Labeling Training Data: The Foundation of Successful Machine Learning
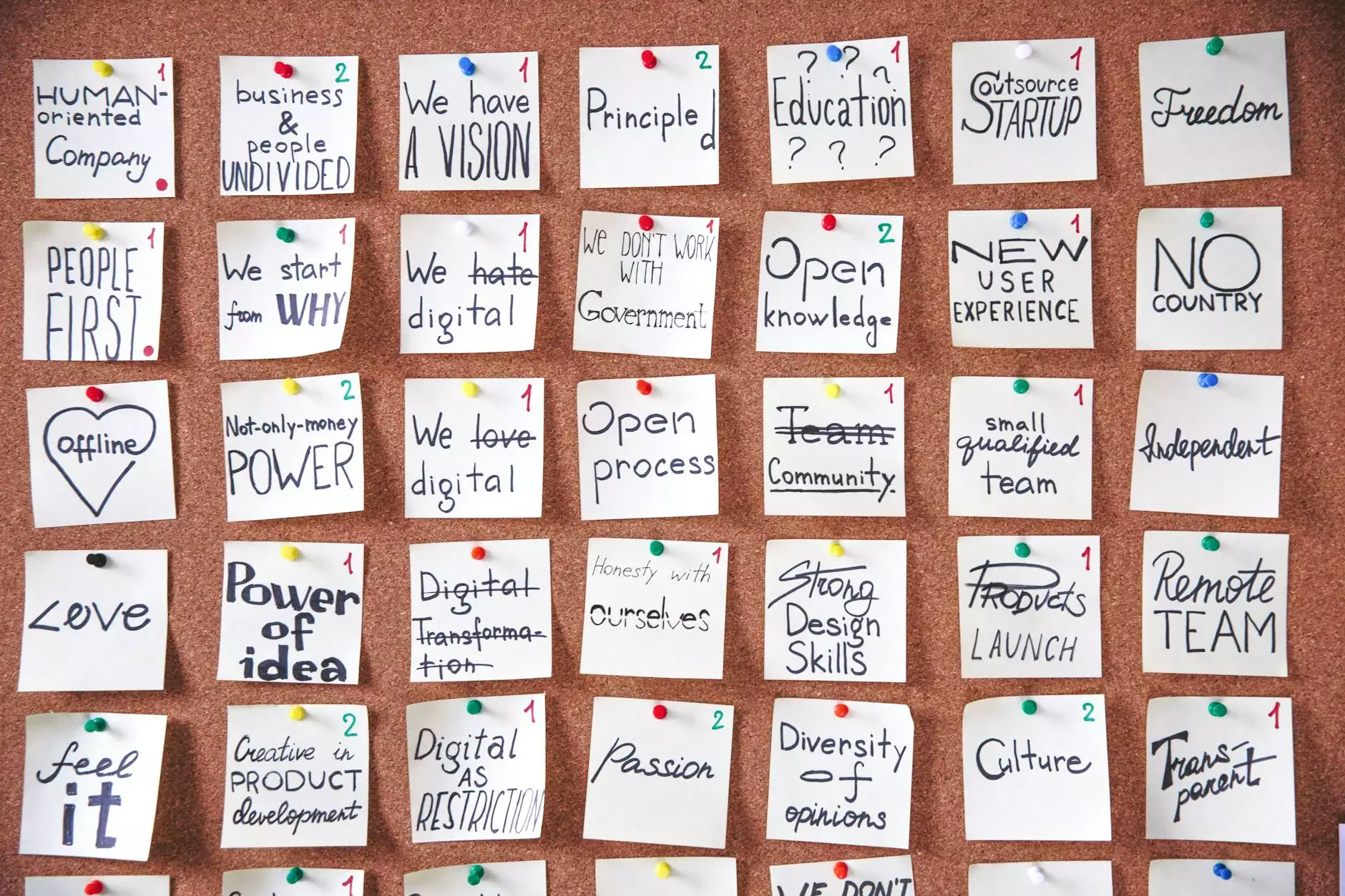
In today's data-driven world, the success of machine learning applications hinges significantly on the availability of high-quality, labeled training data. As organizations increasingly turn to artificial intelligence (AI) to drive innovation, understanding the critical role of data labeling becomes paramount for developers, data scientists, and business leaders alike.
Understanding the Significance of Data Annotation
Data annotation, synonymous with data labeling, is the process of categorizing and tagging data to make it understandable for machine learning algorithms. This process is essential as algorithms learn from labeled data to make predictive analyses or decisions based on new input. Without proper labeling, even the most sophisticated algorithms will struggle to perform effectively.
Why Labeling Training Data is Crucial
The significance of labeling training data can be encapsulated in three crucial points:
- Accuracy: Labeled data ensures that models are trained on correct and precise information, thereby improving prediction accuracy.
- Efficiency: Well-labeled data allows for quick training of machine learning models, saving time and resources.
- Scalability: High-quality labeled datasets facilitate the scaling of machine learning solutions, enabling them to adapt to new challenges and larger datasets.
The Challenges of Data Labeling
Despite its importance, the process of labeling training data can be fraught with challenges, such as:
Subjectivity and Consistency
One of the primary challenges in data annotation is achieving consistency in labeling. Different annotators might apply their interpretations, leading to discrepancies in the data. To tackle this, leveraging a robust data annotation platform like Key Labs is essential.
Time Consumption
Labeling massive datasets can be time-consuming, especially when performed manually. Automation and effective tools can significantly reduce the time taken for this essential process.
Handling Complex Data
As datasets become more complex, comprising various data types such as text, audio, images, and videos, the challenges of labeling increase. Accurate annotation tools must be capable of handling these complexities.
Introducing Key Labs: Your Partner in Data Annotation
Key Labs offers cutting-edge solutions for dealing with the challenges associated with labeling training data. With our robust data annotation platform, we provide businesses with the tools necessary to ensure data accuracy and efficiency.
Features of Key Labs' Data Annotation Tool
Our data annotation tools come equipped with numerous features tailored to enhance your machine learning projects:
- Automation: Leverage AI-assisted tools that speed up the labeling process while maintaining high accuracy.
- User-Friendly Interface: Intuitive design that allows both technical and non-technical users to navigate easily.
- Customizable Solutions: Tailor annotation projects to meet specific business needs and requirements.
The Benefits of Using Key Labs for Labeling Training Data
Partnering with Key Labs provides several advantages:
Quality Control
We implement strict quality assurance measures to ensure the integrity of labeled data, enhancing the model's learning process. Structured review processes guarantee that the data meets the specifications of your project.
Scalability
As your data needs grow, Key Labs scales with you, providing the capability to handle increasing volumes of data without sacrificing quality or speed.
Expert Guidance
Key Labs boasts a team of experienced annotators who understand the intricacies of effective data labeling. This team ensures that your data is not just labeled but optimized for machine learning success.
Strategies for Effective Labeling Training Data
To maximize the effectiveness of your labeled training data, consider the following strategies:
Define Clear Guidelines
Creating comprehensive guidelines for annotators will enhance consistency and accuracy across large datasets. Detailed instructions reduce ambiguity and lead to improved labeling quality.
Employ Specialized Annotators
In fields where domain knowledge is required, employing specialized annotators can significantly improve the quality of the labeled data. Understanding specific nuances can lead to better data interpretation.
Regular Review and Feedback
Establishing a process for regular reviews and providing feedback to annotators will iterate the quality of their work. Continuous training and improvement will lead to superior labeling outcomes.
Conclusion: The Future of Labeling Training Data
As AI technologies advance, the demand for labeling training data will only increase. Investing in high-quality data annotation methods through platforms like Key Labs can serve as a fundamental step towards achieving successful machine learning outcomes.
Labeling training data effectively is not just an operational necessity; it is the cornerstone of an organization's AI strategies. By utilizing robust tools and adhering to best practices, businesses can ensure they remain competitive in an ever-evolving technological landscape. Explore your data annotation needs with Key Labs today and take your AI projects to unprecedented heights!